Help us protect the commons. Make a tax deductible gift to fund our work in 2025. Donate today!
Exploring Preference Signals for AI Training
Better Internet, Open Culture, Technology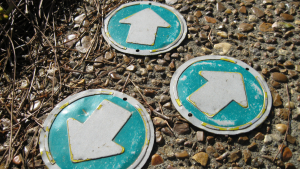
“Choices” by Derek Bruff, here cropped, licensed via CC BY-NC 2.0.
One of the motivations for founding Creative Commons (CC) was offering more choices for people who wish to share their works openly. Through engagement with a wide variety of stakeholders, we heard frustrations with the “all or nothing” choices they seemed to face with copyright. Instead they wanted to let the public share and reuse their works in some ways but not others. We also were motivated to create the CC licenses to support people — artists, technology developers, archivists, researchers, and more — who wished to re-use creative material with clear, easy-to-understand permissions.
What’s more, our engagement revealed that people were motivated to share not merely to serve their own individual interests, but rather because of a sense of societal interest. Many wanted to support and expand the body of knowledge and creativity that people could access and build upon — that is, the commons. Creativity depends on a thriving commons, and expanding choice was a means to that end.
Similar themes came through in our community consultations on generative artificial intelligence (AI*). Obviously, the details of AI and technology in society in 2023 are different from 2002. But the challenges of an all-or-nothing system where works are either open to all uses, including AI training, or entirely closed, are a through-line. So, too, is the desire to do so in a way that supports creativity, collaboration, and the commons.
One option that was continually raised was preference signaling: a way of making requests about some uses, not enforceable through the licenses, but an indication of the creators’ wishes. We agree that this is an important area of exploration. Preference signals raise a number of tricky questions, including how to ensure they are a part of a comprehensive approach to supporting a thriving commons — as opposed to merely a way to limit particular ways people build on existing works, and whether that approach is compatible with the intent of open licensing. At the same time, we do see potential for them to help facilitate better sharing.
What We Learned: Broad Stakeholder Interest in Preference Signals
In our recent posts about our community consultations on generative AI, we have highlighted the wide range of views in our community about generative AI.
Some people are using generative AI to create new works. Others believe it will interfere with their ability to create, share, and earn compensation, and they object to current ways AI is trained on their works without express permission.
While many artists and content creators want clearer ways to signal their preferences for use of their works to train generative AI, their preferences vary. Between the poles of “all” and “nothing,” there were gradations based on how generative AI was used specifically. For instance, they varied based on whether generative AI is used
- to edit a new creative work (similar to the way one might use Photoshop or another editing program to alter an image),
- to create content in the same category of the works it was trained on (i.e., using pictures to generate new pictures),
- to mimic a particular person or replace their work generally, or
- to mimic a particular person and replace their work to commercially pass themselves off as the artist (as opposed to doing a non-commercial homage, or a parody).
Views also varied based on who created and used the AI — whether researchers, nonprofits, or companies, for instance.
Many technology developers and users of AI systems also shared interest in defining better ways to respect creators’ wishes. Put simply, if they could get a clear signal of the creators’ intent with respect to AI training, then they would readily follow it. While they expressed concerns about over-broad requirements, the issue was not all-or-nothing.
Preference Signals: An Ambiguous Relationship to a Thriving Commons
While there was broad interest in better preference signals, there was no clear consensus on how to put them into practice. In fact, there is some tension and some ambiguity when it comes to how these signals could impact the commons.
For example, people brought up how generative AI may impact publishing on the Web. For some, concerns about AI training meant that they would no longer be sharing their works publicly on the Web. Similarly, some were specifically concerned about how this would impact openly licensed content and public interest initiatives; if people can use ChatGPT to get answers gleaned from Wikipedia without ever visiting Wikipedia, will Wikipedia’s commons of information continue to be sustainable?
From this vantage point, the introduction of preference signals could be seen as a way to sustain and support sharing of material that might otherwise not be shared, allowing new ways to reconcile these tensions.
On the other hand, if preference signals are broadly deployed just to limit this use, it could be a net loss for the commons. These signals may be used in a way that is overly limiting to expression — such as limiting the ability to create art that is inspired by a particular artist or genre, or the ability to get answers from AI systems that draw upon significant areas of human knowledge.
Additionally, CC licenses have resisted restrictions on use, in the same manner as open source software licenses. Such restrictions are often so broad that they cut off many valuable, pro-commons uses in addition to the undesirable uses; generally the possibility of the less desirable uses is a tradeoff for the opportunities opened up by the good ones. If CC is endorsing restrictions in this way we must be clear that our preference is a “commons first” approach.
This tension is not easily reconcilable. Instead, it suggests that preference signals are by themselves not sufficient to help sustain the commons, and should be explored as only a piece of a broader set of paths forward.
Existing Preference Signal Efforts
So far, this post has spoken about preference signals in the abstract, but it’s important to note that there are already many initiatives underway on this topic.
For instance, Spawning.ai has worked on tools to help artists find if their works are contained in the popular LAION-5B dataset, and decide whether or not they want to exclude them. They’ve also created an API that enables AI developers to interoperate with their lists; StabilityAI has already started accepting and incorporating these signals into the data they used to train their tools, respecting artists’ explicit opt-ins and opt-outs. Eligible datasets hosted on the popular site Hugging Face also now show a data report powered by Spawning’s API, informing model trainers what data has been opted out and how to remove it. For web publishers, they’ve also been working on a generator for “ai.txt” files that signals restrictions or permissions for the use of a site’s content for commercial AI training, similar to robots.txt.
There are many other efforts exploring similar ideas. For instance, a group of publishers within the World Wide Web Consortium (W3C) is working on a standard by which websites can express their preferences with respect to text and data mining. The EU’s copyright law expressly allows people to opt-out from text and data mining through machine-readable formats, and the idea is that the standard would fulfill that purpose. Adobe has created a “Do Not Train” metadata tag for works generated with some of its tools, Google has announced work to build an approach similar to robots.txt, and OpenAI has provided a means for sites to exclude themselves from crawling for future versions of GPT.
Challenges and Questions in Implementing Preference Signals
These efforts are still in relatively early stages, and they raise a number of challenges and questions. To name just a few:
- Ease-of-Use and Adoption: For preference signals to be effective, they must be easy for content creators and follow-on users to make use of. How can solutions be ease-to-use, scalable, and accommodate different types of works, uses, and users?
- Authenticating Choices: How best to validate and trust that a signal has been put in place by the appropriate party? Relatedly, who should be able to set the preferences — the rightsholder for the work, the artist who originally created it, both?
- Granular Choices for Artists: So far, most efforts have been focused on enabling people to opt-out of use for AI training. But as we note above, people have a wide variety of preferences, and preference signals should also be a way for people to signal that they are OK with their works being used, too. How might signals strike the right balance, enabling people to express granular preferences, but without becoming too cumbersome
- Tailoring and Flexibility Based on Types of Works and Users: We’ve focused in this post on artists, but there are of course a wide variety of types of creators and works. How can preference signals accommodate scientific research, for instance? In the context of indexing websites, commercial search engines generally follow the robots.txt protocol, although institutions like archivists and cultural heritage organizations may still crawl to fulfill their public interest missions. How might we facilitate similar sorts of norms around AI?
As efforts to build preference signals continue, we will continue to explore these and other questions in hopes of informing useful paths forward. Moreover, we will also continue to explore other mechanisms necessary to help support sharing and the commons. CC is committed to more deeply engaging in this subject, including at our Summit in October, whose theme is “AI and the Commons.”
If you are in New York City on 13 September 2023, join our symposium on Generative AI & the Creativity Cycle, which focuses on the intersection of generative artificial intelligence, cultural heritage, and contemporary creativity. If you miss the live gathering, look for the recorded sessions.
Like the rest of the world, CC has been watching generative AI and trying to understand the many complex issues raised by these amazing new tools. We are especially focused on the intersection of copyright law and generative AI. How can CC’s strategy for better sharing support the development of this technology while also respecting the work of human creators? How can we ensure AI operates in a better internet for everyone? We are exploring these issues in a series of blog posts by the CC team and invited guests that look at concerns related to AI inputs (training data), AI outputs (works created by AI tools), and the ways that people use AI. Read our overview on generative AI or see all our posts on AI.
Note: We use “artificial intelligence” and “AI” as shorthand terms for what we know is a complex field of technologies and practices, currently involving machine learning and large language models (LLMs). Using the abbreviation “AI” is handy, but not ideal, because we recognize that AI is not really “artificial” (in that AI is created and used by humans), nor “intelligent” (at least in the way we think of human intelligence).